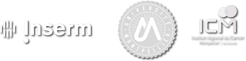
Added by | standudu |
---|---|
Group name | EquipeCTCS |
Item Type | Journal Article |
Title | Machine Learning-Assisted Evaluation of Circulating DNA Quantitative Analysis for Cancer Screening |
Creator | Tanos et al. |
Author | Rita Tanos |
Author | Guillaume Tosato |
Author | Zahra Al Amir Dache |
Author | Laurence Pique Lasorsa |
Author | Geoffroy Tousch |
Author | Safia El Messaoudi |
Author | Romain Meddeb |
Author | Mona Diab Assaf |
Author | Marc Ychou |
Author | Denis Pezet |
Author | Johan Gagnière |
Author | Pierre-Emmanuel Colombo |
Author | William Jacot |
Author | Marie Dupuy |
Author | Antoine Adenis |
Author | Thibault Mazard |
Author | Caroline Mollevi |
Author | José María Sayagués |
Author | Jacques Colinge |
Author | Alain R. Thierry |
Abstract | While the utility of circulating cell-free DNA (cfDNA) in cancer screening and early detection have recently been investigated by testing genetic and epigenetic alterations, here, an original approach by examining cfDNA quantitative and structural features is developed. First, the potential of cfDNA quantitative and structural parameters is independently demonstrated in cell culture, murine, and human plasma models. Subsequently, these variables are evaluated in a large retrospective cohort of 289 healthy individuals and 983 patients with various cancer types; after age resampling, this evaluation is done independently and the variables are combined using a machine learning approach. Implementation of a decision tree prediction model for the detection and classification of healthy and cancer patients shows unprecedented performance for 0, I, and II colorectal cancer stages (specificity, 0.89 and sensitivity, 0.72). Consequently, the methodological proof of concept of using both quantitative and structural biomarkers, and classification with a machine learning method are highlighted, as an efficient strategy for cancer screening. It is foreseen that the classification rate may even be improved by the addition of such biomarkers to fragmentomics, methylation, or the detection of genetic alterations. The optimization of such a multianalyte strategy with this machine learning method is therefore warranted. |
Publication | Advanced Science (Weinheim, Baden-Wurttemberg, Germany) |
Volume | 7 |
Issue | 18 |
Pages | 2000486 |
Date | 2020-09 |
Journal Abbr | Adv Sci (Weinh) |
Language | eng |
DOI | 10.1002/advs.202000486 |
ISSN | 2198-3844 |
Library Catalog | PubMed |
Extra | PMID: 32999827 PMCID: PMC7509651 |
Tags | cancer, machine learning, original, screening |
Date Added | 2023/11/14 - 15:16:39 |
Date Modified | 2023/11/14 - 15:47:17 |
Notes and Attachments | PubMed entry (Attachment) Texte intégral (Attachment) |